Vertex AI is a Google Cloud platform designed to streamline the entire Machine Learning (ML) lifecycle, from data preparation to deployment and monitoring [4]. It essentially provides a unified environment for you to train and use ML models and various AI applications.
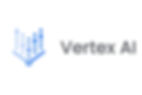
Here's a breakdown of Vertex AI and how you can leverage it:
What it Does:
Manages ML Workflow:
Vertex AI simplifies the complex stages of building ML models into a manageable sequence. This includes prepping data, training models, deploying them, and overseeing their performance.
Complex Stages Made Simpler:
Imagine you're building a machine learning model to predict if a customer will churn (cancel their service). Traditionally, this would involve:
Data Wrangling: Manually collecting customer data from various sources, cleaning it for inconsistencies, and formatting it for the model.
Model Training: Choosing an algorithm, writing code to train the model on the prepared data, and fine-tuning its parameters for optimal performance.
Deployment: Setting up servers, deploying the model to make predictions, and integrating it with your application.
Monitoring: Tracking the model's performance over time, identifying any accuracy drops, and potentially retraining it with new data.
Vertex AI to the Rescue:
Vertex AI simplifies these steps significantly:
Data Prep:
Easy Ingestion: Connect Vertex AI to your customer data sources (databases, spreadsheets etc.) and import the data seamlessly.
Built-in Cleaning Tools: Utilize Vertex AI's tools to address missing data, normalize values (like income ranges), and automatically generate relevant features for your model (e.g., customer tenure).
Model Training:
AutoML for Efficiency: If you're new to ML, leverage Vertex AI's AutoML. You simply provide your data and choose the desired outcome (predict churn in this case). AutoML explores various algorithms, identifies the best fit, and trains the model automatically.
Customizable Training (Optional): For experienced data scientists, Vertex AI offers complete control. You can choose specific algorithms, write your own training code using frameworks like TensorFlow, and fine-tune the model's hyperparameters for optimal performance.
Deployment made Easy:
Flexible Deployment Options: Deploy your churn prediction model as a web service within Vertex AI. This allows your application to send customer data to the model in real-time and receive churn probability predictions.
Automatic Scaling: No need to worry about server capacity. Vertex AI automatically scales the underlying infrastructure to handle increasing prediction requests, ensuring smooth operation.
Monitoring for Continuous Improvement:
Performance Tracking: Vertex AI continuously monitors your deployed model's accuracy in predicting churn. You can see metrics like how often the model correctly identifies customers at risk of churning.
Collaboration:
Data scientists, developers, and other users can work together on projects using a common set of tools within Vertex AI.
Collaboration Made Easy with Vertex AI
Traditionally, building machine learning (ML) models involved specialists working in silos. Data scientists prepared the data, developers built the models, and others managed deployment and monitoring. This could lead to inefficiencies and communication gaps.
Vertex AI tackles this challenge by fostering collaboration through a common set of tools accessible to various users:
Centralized Platform:
Shared Workspace: Data scientists, developers, and other users can all work within the same Vertex AI environment. This eliminates the need for multiple tools or data copies, promoting a unified approach.
Version Control: Vertex AI allows version control for data, models, and experiments. This ensures everyone is working on the latest version and facilitates tracking changes and rollbacks if needed.
Unified Toolset:
Standardized Interfaces: Vertex AI provides user-friendly interfaces for different roles. Data scientists can leverage tools for data exploration and feature engineering, while developers can access APIs and libraries for model deployment and integration. Non-technical users might utilize dashboards for model monitoring.
Streamlined Workflows: The platform offers predefined workflows for common ML tasks like data preparation, training, and deployment. This ensures everyone follows a consistent approach and avoids errors or inefficiencies.
Communication and Sharing:
Documentation and Notes: Vertex AI allows users to add documentation and notes to data, models, and experiments. This fosters communication and knowledge sharing within the team, making it easier for everyone to understand the project's context and rationale.
Collaboration Features: Some features, like Vertex Notebooks, provide a shared environment where team members can work together on code, data exploration, and model development in real-time.
Benefits of Collaboration:
Improved Efficiency: By working together on a centralized platform, teams can streamline workflows, reduce communication overhead, and accelerate project completion.
Enhanced Innovation: Collaboration allows for diverse perspectives and expertise to be combined, leading to more creative solutions and better performing ML models.
Reduced Errors: A common set of tools and standardized workflows minimize the risk of errors due to miscommunication or incompatible formats.
Overall, Vertex AI fosters collaboration by providing a central platform, a unified toolset, and communication features that streamline workflows, boost innovation, and reduce errors within your ML projects.
Automates Tasks (AutoML)
For those new to ML, Vertex AI offers AutoML which automates many tasks involved in model creation, reducing the need for extensive coding.
Machine learning (ML) offers incredible capabilities, but for those new to the field, building models can seem complex and require extensive coding expertise. Vertex AI tackles this barrier with AutoML, a suite of tools that automates many tasks involved in model creation.
How AutoML Works:
Simplified Workflow: Instead of writing complex code for each stage of model development, AutoML provides a user-friendly interface. You simply:
Provide Your Data: Upload your training data to Vertex AI.
Choose Your Goal: Specify the desired outcome for your model (e.g., image classification, text sentiment analysis, predicting customer churn).
Let AutoML Take Over: Vertex AI analyzes your data, explores different algorithms, and automatically trains the model best suited for your task.
Reduced Coding Needs: AutoML handles tasks like feature engineering (preparing data for the model), hyperparameter tuning (optimizing the model's performance), and even model selection. This significantly reduces the need for extensive coding knowledge.
Benefits of AutoML:
Faster Development: By automating many steps, AutoML streamlines the model creation process, allowing you to build and deploy models quicker.
Accessibility for Beginners: Those new to ML can leverage AutoML to create effective models without needing to be coding experts.
Exploration and Experimentation: AutoML allows you to easily experiment with different algorithms and data sets to find the best solution for your specific needs.
Here's an example:
Imagine you want to build a model to classify images based on content (e.g., identifying cats in pictures). Traditionally, you'd need to write code to prepare the image data, choose and configure an appropriate algorithm, and then train the model.
With Vertex AI's AutoML for Image Classification, you simply upload your image dataset and specify that you want to classify image content. AutoML takes care of the rest, automatically training a model that can effectively categorize your images.
Important Note:
While AutoML is powerful, it may not always be the best choice for complex tasks or highly customized models. For experienced data scientists, Vertex AI also offers options for custom model development using frameworks like TensorFlow.
In conclusion, Vertex AI's AutoML empowers those new to ML to build effective models, while also offering flexibility for experienced users. This democratizes machine learning, making its potential accessible to a wider range of users.
Scalability
The platform can handle large datasets and complex models without you having to worry about managing servers. Google Cloud takes care of the underlying infrastructure.
Generative AI: You can even build and use generative AI models through Vertex AI, which can create new content like text, code, or images.
Vertex AI offers two key functionalities beyond the aspects we've discussed so far:
1. Scalability:
Handles Large Datasets and Complex Models: Traditional ML tools can struggle with massive datasets or intricate models. Vertex AI solves this by leveraging Google Cloud's robust infrastructure. You can train and deploy complex models on ever-growing datasets without worrying about server limitations.
Automatic Scaling: Vertex AI automatically scales the underlying compute resources based on your needs. This ensures smooth operation even during spikes in prediction requests. You don't need to manually manage servers or worry about infrastructure bottlenecks.
Benefits of Scalability:
Tackle Ambitious Projects: The ability to handle large datasets opens doors to tackling ambitious ML projects that wouldn't be feasible with traditional tools.
Cost-Effectiveness: Automatic scaling ensures you only pay for the resources you use. This avoids paying for excess server capacity during low-demand periods.
Focus on Innovation: By eliminating infrastructure concerns, Vertex AI allows you to focus on developing innovative ML models and solutions.
2. Generative AI:
Build and Use Generative Models: Vertex AI goes beyond traditional predictive models. It allows you to build and use generative AI models, a powerful new technology.
Create New Content: Generative AI models can create entirely new content, like realistic images, creative text formats (poems, code), or different variations of existing data.
Examples of Generative AI with Vertex AI:
Generate Product Descriptions: An e-commerce company could use a generative model to create unique and engaging product descriptions based on existing data.
Develop Creative Marketing Content: A marketing team could leverage generative AI to create variations of ad copy or social media posts, optimizing for different audiences.
Personalize User Experiences: An application could use a generative model to personalize content recommendations or product suggestions for each user.
Generative AI represents a new frontier in machine learning, and Vertex AI empowers you to leverage this potential.
By combining scalability and generative AI capabilities, Vertex AI offers a powerful platform for tackling complex ML challenges and exploring innovative solutions.
How to Use It:
Getting Started: Google Cloud offers a comprehensive guide to get you started with Vertex AI, including an introduction to its functionalities.
Tutorials: There are tutorials available that walk you through specific tasks like training regression or classification models for various data types (tabular data, video) using Vertex AI and AutoML. You can choose between Cloud Console or Jupyter Notebook for these tutorials .
Now that you understand the core functionalities of Vertex AI, let's explore how to get started using it:
1. Getting Started with Vertex AI:
Google Cloud Guide: Google Cloud offers a comprehensive guide specifically designed to help you get started with Vertex AI. This guide includes:
An introduction to Vertex AI's functionalities and key features.
Step-by-step instructions on setting up your Vertex AI environment.
Resources and documentation to help you navigate the platform.
Here's where to find the guide: "https://cloud.google.com/vertex-ai"
2. Tutorials for Hands-on Learning:
Vertex AI Tutorials: Google Cloud provides a collection of tutorials specifically designed to guide you through common ML tasks using Vertex AI and AutoML. These tutorials cover various data types and tasks, including:
Training regression or classification models for tabular data (structured data in tables).
Building models for video or image data.
Choose Your Learning Environment: The tutorials offer flexibility in how you learn. You can choose to work in either:
Cloud Console: A web-based interface that provides a user-friendly environment for interacting with Vertex AI features.
Jupyter Notebook: A popular interactive coding environment commonly used for data science tasks. This allows you to experiment with code and data within the tutorials.
Benefits of Tutorials:
Practical Learning: The tutorials provide a hands-on approach to learning Vertex AI. You can follow the steps, build your own models, and gain practical experience with the platform.
Variety of Topics: With tutorials covering different data types and tasks, you can find ones that cater to your specific interests and goals.
Flexible Learning Environment: The option to choose between Cloud Console or Jupyter Notebook allows you to learn in a way that suits your comfort level and technical background.
By leveraging the getting started guide and tutorials, you can gain a solid foundation in using Vertex AI to build and deploy your own machine learning models.