ChatGPT is a language model developed by OpenAI. It is based on the GPT (Generative Pre-trained Transformer) architecture, specifically GPT-3.5. The model has been trained on a large amount of text data from the internet to understand and generate human-like text responses.
Here's a The Secret of ChatGpt got Revealed
Training Data:
ChatGPT is trained on a vast amount of text data from various sources like books, articles, websites, and other publicly available written material. The training data helps the model learn the patterns, grammar, and contextual information in the text.
The training data used for ChatGPT consists of a diverse range of text from various sources. Here are some details about the training data and how it helps the model learn:
Books: ChatGPT is trained on a large corpus of books covering a wide array of genres, topics, and writing styles. This includes both fiction and non-fiction books, providing a rich source of textual information. Books help the model learn grammar, vocabulary, sentence structures, and storytelling techniques.
Articles: The model is exposed to a vast collection of articles from newspapers, magazines, journals, and other publications. This includes news articles, opinion pieces, scientific papers, and more. By training on articles, the model learns about current events, factual information, writing conventions, and different styles of journalistic writing.
Websites: ChatGPT is trained on a diverse set of websites that cover a wide range of topics. This includes general knowledge websites, educational resources, forums, blogs, and more. Training on website text exposes the model to a variety of writing styles, domain-specific knowledge, and informal language usage.
Publicly Available Text: The training data may also include other publicly available written material such as publicly accessible parts of the internet, online forums, open-access repositories, and similar sources. This ensures that the model is exposed to a wide range of text from different contexts and perspectives.
By training on such diverse and extensive text data, ChatGPT learns to recognize patterns, understand grammar rules, capture contextual information, and generate text that aligns with human-like language usage. It helps the model develop a broad understanding of language, common knowledge, and writing conventions present in the training data.
However, it's important to note that the training data is a reflection of the content available on the internet, which can include biases and inaccuracies. OpenAI has taken steps to address biases during the fine-tuning process and continues to work on improving the model's performance and mitigating potential issues.
Transformer Architecture:
GPT models use a deep learning architecture known as the Transformer. Transformers are designed to handle sequential data efficiently, and they excel at capturing relationships between words in a sentence.
The Transformer architecture is a deep learning model architecture that was introduced in the paper "Attention Is All You Need" by Vaswani et al. (2017).
It revolutionized natural language processing tasks, including language translation, summarization, and generation tasks like ChatGPT. Here are the key components and concepts of the Transformer architecture:
Self-Attention Mechanism:
The Transformer model utilizes a self-attention mechanism, also known as scaled dot-product attention. This mechanism allows the model to weigh the importance of different words or tokens in a sentence when generating a representation for each word. Self-attention captures dependencies between words by attending to other words in the same sentence and learning the relevance of each word to the others. It helps the model capture long-range dependencies and contextual relationships between words efficiently.
The self-attention mechanism in the Transformer architecture plays a crucial role in capturing dependencies between words in a sentence. Let's delve into the details:
Key, Query, and Value: The self-attention mechanism operates on a set of key, query, and value vectors. These vectors are derived from the input sequence of words or tokens. For each word in the sequence, a key, a query, and a value vector are generated. These vectors allow the model to compute the relevance and importance of each word with respect to the others.
Scaled Dot-Product Attention: The attention mechanism calculates the attention weights by computing the dot product between the query vector of a given word and the key vectors of all other words in the sequence. The dot products are then scaled by the square root of the dimensionality of the key vectors to prevent the gradients from becoming too large.
Attention Weights and Context Vectors: The dot products between the query and key vectors represent the compatibility or similarity between the words. Softmax is applied to these dot products to obtain attention weights that sum up to one. These attention weights reflect the importance of each word in the sequence with respect to the current word. The attention weights are then used to compute weighted sums of the value vectors, yielding the context vectors.
Capturing Dependencies and Relationships: By attending to all other words in the sequence, the self-attention mechanism captures the dependencies and relationships between words. Each word in the sequence can gather information from other words, learn their relevance, and generate a context vector that combines the information from the entire sentence.
Multiple Attention Heads: In the Transformer architecture, the self-attention mechanism is typically applied multiple times in parallel, each with its own set of learned parameters called attention heads. Multiple attention heads allow the model to attend to different positions and learn different patterns within the input sequence. The outputs of the attention heads are concatenated or averaged to produce the final representation for each word.
Positional Encoding: Since the self-attention mechanism doesn't inherently account for word order, positional encoding is used to inject information about the position of each word in the input sequence. Positional encoding helps the model distinguish between words based on their position, enabling it to capture the sequential relationships and contextual dependencies.
By employing the self-attention mechanism, the Transformer model can effectively weigh the importance of different words in a sentence, capture long-range dependencies, and generate contextual representations for each word based on its relationships with other words in the sequence. This enables the model to generate more accurate and contextually relevant responses in natural language processing tasks.
Encoder-Decoder Structure: The Transformer architecture consists of an encoder and a decoder. The encoder processes the input sequence, such as the question or prompt, while the decoder generates the output sequence, such as the model's response. The self-attention mechanism is employed in both the encoder and decoder to capture relationships within the input and output sequences.
Multi-Head Attention: The self-attention mechanism is applied multiple times in parallel, each with its own set of learned parameters called attention heads. Multiple attention heads allow the model to attend to different positions and capture different types of dependencies simultaneously. This multi-head attention mechanism helps the model capture various types of information and learn different patterns within the input sequence.
Positional Encoding: Since Transformer models don't have an inherent notion of word order, positional encoding is used to inject information about the position of each word in the input sequence. Positional encoding is a vector representation that carries positional information and is added to the word embeddings. This enables the model to differentiate between words based on their position in the sequence.
Feed-Forward Neural Networks: Transformers employ feed-forward neural networks within each layer of the encoder and decoder. These networks apply non-linear transformations to the representations learned from the self-attention mechanism, enabling the model to capture more complex relationships and patterns in the data.
Layer Normalization: Layer normalization is applied after each sub-layer in the Transformer model. It normalizes the inputs to each layer, helping to stabilize the learning process and improve the overall performance of the model.
By utilizing the Transformer architecture, GPT models like ChatGPT are able to efficiently process and understand the relationships between words in a sentence. The self-attention mechanism, multi-head attention, positional encoding, and feed-forward networks all contribute to the model's ability to generate coherent and contextually relevant responses. The Transformer architecture has been widely adopted and has achieved state-of-the-art performance in various natural language processing tasks.
Pre-training:
Before it can be used for chat interactions, the model undergoes a pre-training phase. During pre-training, the model learns to predict the next word in a sentence based on the context of previous words. This process helps the model develop an understanding of language and its nuances.
The encoder-decoder structure is a fundamental component of the Transformer architecture. It involves two main components: the encoder and the decoder. Let's explore how they work together:
Encoder: The encoder processes the input sequence, such as a question or prompt, and generates a contextual representation of the input. It consists of multiple layers, each containing sub-layers, such as self-attention and feed-forward neural networks. Here's an overview of how the encoder works:
Input Embedding: The input sequence is initially transformed into a sequence of continuous vector representations known as word embeddings. These embeddings capture the semantic meaning and syntactic properties of the words in the input.
Positional Encoding: Since the Transformer architecture doesn't inherently understand word order, positional encoding is added to the word embeddings. This allows the model to differentiate between words based on their positions in the sequence.
Self-Attention Layers: The self-attention mechanism is applied in multiple layers of the encoder. Each self-attention layer attends to all other words in the input sequence to capture dependencies and relationships between words. The outputs of the self-attention layers contain contextual information about each word in the input.
Feed-Forward Networks: After the self-attention layers, a feed-forward neural network is applied to the outputs of each self-attention layer. This network applies non-linear transformations to further process and refine the representations of each word.
Layer Normalization: Layer normalization is applied after each sub-layer in the encoder. It normalizes the inputs to each layer, helping to stabilize the learning process and improve the overall performance of the model.
The final output of the encoder is a sequence of contextual representations for each word in the input sequence. These representations capture the relationships, contextual dependencies, and semantic information present in the input.
Decoder: The decoder generates the output sequence based on the contextual representations generated by the encoder. It also consists of multiple layers, including self-attention and cross-attention sub-layers. Here's an overview of how the decoder works:
Target Embedding: The decoder takes as input a target sequence, such as the model's response or output. Similar to the encoder, the target sequence is transformed into word embeddings.
Positional Encoding: The positional encoding is added to the target embeddings to provide information about the position of each word in the sequence.
Self-Attention Layers: Similar to the encoder, self-attention layers are applied in the decoder. These layers attend to other words in the target sequence, allowing the decoder to capture dependencies and relationships between words in the output.
Encoder-Decoder Attention: In addition to self-attention, the decoder also employs cross-attention layers that attend to the contextual representations generated by the encoder. This enables the decoder to focus on relevant parts of the input sequence when generating the output.
Feed-Forward Networks: After the self-attention and cross-attention layers, feed-forward neural networks are applied to process and refine the representations of each word in the target sequence.
Layer Normalization: Similar to the encoder, layer normalization is applied after each sub-layer in the decoder to improve stability and performance.
The decoder generates a sequence of contextual representations for each word in the target sequence, capturing the relationships between words and their dependencies on the input.
By employing the encoder-decoder structure, the Transformer architecture enables the model to process the input sequence, capture relationships and dependencies, and generate a contextually appropriate output sequence based on the learned representations. The self-attention mechanism plays a key role in both the encoder and decoder, allowing the model to effectively attend to relevant parts of the input and output sequences.
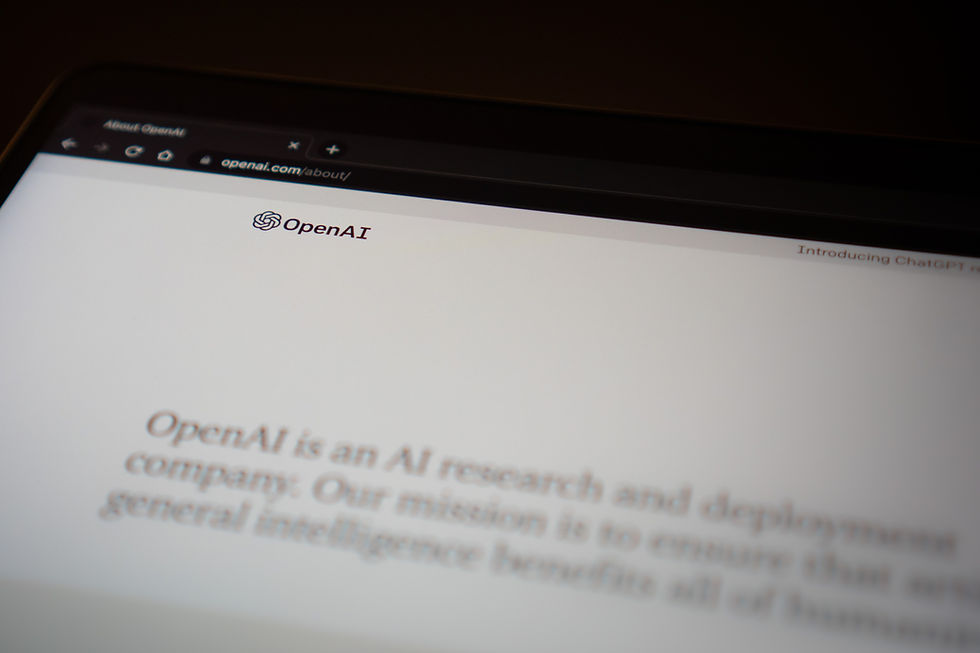
Fine-tuning:
After pre-training, the model goes through a fine-tuning process. In this phase, the model is further trained on a more specific dataset that is carefully generated with the help of human reviewers. These reviewers follow guidelines provided by OpenAI to review and rate potential model outputs. The fine-tuning process helps align the model's responses with human values and improves its overall performance.
Fine-tuning is a critical phase in training models like ChatGPT to align their responses with human values and improve their overall performance. Here's a detailed explanation of the fine-tuning process:
Initial Pre-training: ChatGPT models are initially pre-trained on a large corpus of publicly available text from various sources, as mentioned earlier. During this pre-training phase, the model learns to predict the next word in a sentence based on the context of previous words. This process helps the model develop an understanding of language and its patterns.
Dataset Creation: To fine-tune the model, a more specific dataset is created. OpenAI works with human reviewers to generate this dataset. The reviewers follow guidelines provided by OpenAI to review and rate potential model outputs. The dataset is carefully crafted to ensure high-quality and safe training examples.
Guidelines and Review Process: OpenAI provides guidelines to the human reviewers, which include instructions on desired behavior, potential pitfalls, and potential issues to avoid. These guidelines aim to improve the alignment of the model's responses with human values and mitigate any potential biases or concerns.
Iteration and Feedback: The fine-tuning process is iterative, involving a feedback loop between OpenAI and the reviewers. OpenAI maintains a strong feedback relationship with the reviewers, engaging in regular meetings to address questions, provide clarifications, and discuss challenges. This iterative process helps improve the model's performance over time.
Model Adaptation: The fine-tuning process involves training the model on the specific dataset created with the help of human reviewers. This dataset consists of example inputs and corresponding desired outputs, allowing the model to adapt to the desired behavior and values. The model's parameters are adjusted during fine-tuning to optimize its performance on this dataset.
Performance Monitoring and Iterative Refinement: Throughout the fine-tuning process, OpenAI closely monitors the model's performance, gathers feedback from users, and incorporates ongoing research findings. This iterative refinement helps address any issues, biases, or limitations and continuously improves the model's performance and reliability.
By involving human reviewers and carefully curating a dataset with specific guidelines, the fine-tuning process aims to improve the model's responses, align them with human values, and address concerns regarding safety, bias, and other potential issues. OpenAI values user feedback and works to iteratively enhance the model based on ongoing research and real-world usage
Input and Output:
When you interact with ChatGPT, you provide an input prompt or question. The model then processes the input, applies its knowledge and learned patterns, and generates a text response based on that input. The response is generated using a combination of the training data, pre-training, and fine-tuning knowledge.
Here's a detailed explanation of how the input and output process works when interacting with ChatGPT:
Input Prompt: When you interact with ChatGPT, you provide an input prompt or question. This input prompt can be a sentence, a paragraph, or a more extended piece of text that conveys the context or information you want to communicate to the model. The input prompt serves as a starting point for generating the model's response.
Processing the Input: The model processes the input prompt by tokenizing it into smaller units called tokens. Tokens can represent individual words, subwords, or even characters, depending on the specific tokenization scheme used. The tokenization process allows the model to analyze and understand the input at a more granular level.
Knowledge and Learned Patterns: The model applies its knowledge and learned patterns to the tokenized input. This knowledge comes from a combination of sources:
Training Data: The model has been trained on a vast amount of text data from sources like books, articles, websites, and other publicly available written material. It learns patterns, grammar, contextual information, and common knowledge from this training data.
Pre-training: Before fine-tuning, the model undergoes a pre-training phase where it learns to predict the next word in a sentence based on the context of previous words. This process helps the model develop an understanding of language and its nuances.
Fine-tuning: During the fine-tuning process, the model is trained on a more specific dataset that is generated with the help of human reviewers. This dataset helps align the model's responses with human values and improve its overall performance in terms of generating safe, accurate, and contextually appropriate responses.
Generating the Response: Based on the processed input and its knowledge, the model generates a text response. It employs a combination of techniques, including the self-attention mechanism, transformer architecture, and learned patterns, to generate coherent and contextually relevant responses. The response is generated word by word, with each word influenced by the preceding context and the model's learned patterns.
Output Text: The generated response is then converted back from tokens to human-readable text format. The model produces a text output that represents its response to the input prompt you provided.
It's important to note that while ChatGPT generates responses based on its training and fine-tuning, it does not possess true understanding or consciousness. The model generates responses based on patterns it has learned and does not have real-world knowledge beyond its training data. Additionally, the model's responses can sometimes be creative but may also exhibit limitations or provide inaccurate information, so it's always advisable to verify critical or factual information from reliable sources.
Iterative Refinement:
OpenAI iteratively refines and improves the model based on user feedback and ongoing research. This helps in addressing biases, improving performance, and making the model more reliable and useful over time.
Iterative refinement is a crucial process employed by OpenAI to continually improve the model based on user feedback and ongoing research. It involves several steps to address biases, enhance performance, and make the model more reliable and useful. Here's a detailed explanation of how iterative refinement works:
User Feedback Collection: OpenAI actively seeks and collects user feedback on the model's responses. This feedback can come from users directly interacting with ChatGPT or from external evaluations and studies. User feedback provides valuable insights into the model's strengths, weaknesses, and potential issues.
Identifying Biases and Limitations: OpenAI analyzes user feedback and conducts rigorous evaluations to identify biases, limitations, and areas for improvement in the model's performance. Biases can stem from the training data or the fine-tuning process and may include issues related to race, gender, religion, and more. OpenAI takes these concerns seriously and works towards mitigating biases and addressing limitations.
Research and Development: OpenAI invests in ongoing research and development efforts to advance the capabilities and understand the limitations of the model. This involves conducting studies, exploring novel techniques, and leveraging state-of-the-art advancements in natural language processing. Research and development help drive improvements in the model's performance, reliability, and safety.
Updating Guidelines: OpenAI collaborates with human reviewers and continuously refines the guidelines provided to them. These guidelines ensure that the reviewers can assess and rate potential model outputs effectively. The iterative nature of the process allows OpenAI to address concerns, provide clarifications, and align the model's responses with human values.
Model Updates and Releases: Based on the insights gained from user feedback, research, and guideline updates, OpenAI periodically releases new versions of the model. These updates can include bug fixes, performance improvements, enhancements in response quality, and adjustments to address biases and limitations. Model updates aim to provide users with a more reliable, safe, and useful experience.
Monitoring and User Feedback Loop: After each model release, OpenAI closely monitors user interactions and gathers feedback on the updated model. This feedback loop helps identify any new issues, assess the impact of the updates, and gather further insights for future iterations. OpenAI values user feedback as a valuable resource for improving the model's performance and addressing potential concerns.
The iterative refinement process enables OpenAI to continually enhance the model, address biases, and improve its overall performance and reliability over time. OpenAI's commitment to ongoing research, user feedback, and the collaboration with human reviewers helps create a model that is better aligned with human values and provides a more beneficial experience for users.
It's important to note that while ChatGPT can generate coherent and contextually relevant responses, it does not possess true understanding or consciousness. It generates responses based on patterns it has learned from the training data and does not have real-world knowledge beyond its training. Hope now you can understand what is the Secret of ChatGpt got Revealed.
Comentarios