Making AI Understandable: Solving the Black Box Mystery
- Sandhya Dwivedi
- 19 hours ago
- 4 min read
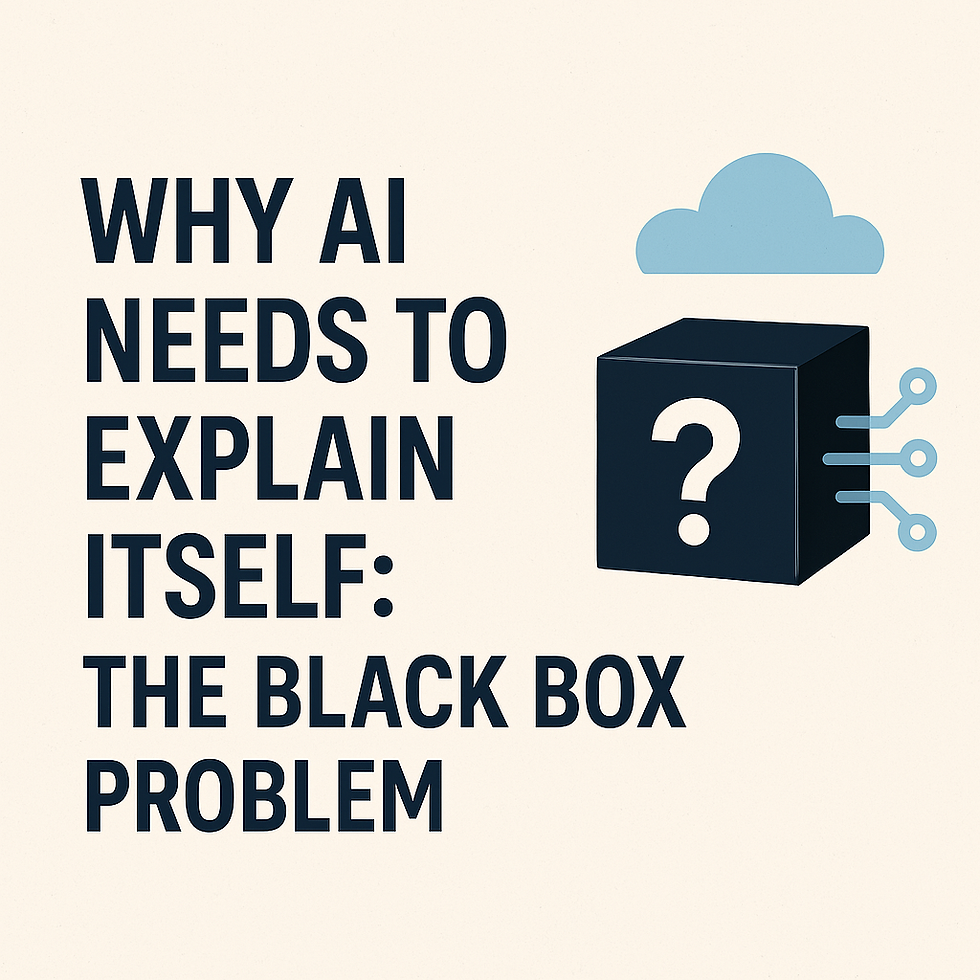
Have You Ever Wondered How AI Thinks?
Imagine a world where AI decides who gets a loan, which candidate gets a job, or even who receives medical treatment—all without explaining why. Sounds unsettling, right?
This is exactly the challenge we face today. AI models, especially deep learning systems, often function as “black boxes”—they make predictions, but even their creators don’t fully understand how they reach conclusions.
So, can we crack open the black box and make AI more transparent? Let’s dive in.
What Is the Black Box Problem?
Think of AI like a magic trick. You see the magician’s final act, but you have no clue how they did it. AI works similarly—it gives results, but the process inside is hidden.
For example:
AI in hospitals predicts diseases but doesn’t explain why.
AI in hiring filters candidates, but we don’t know the criteria.
AI in finance decides who gets a loan, but the logic remains unclear.
This lack of transparency can lead to bias, unfairness, and even mistakes.
The “Black Box” Problem: Why Can’t We See Inside?
To understand the black box problem, let’s compare AI to something more familiar:
Think of a calculator. You input numbers, and it gives you a result. But you can easily track how it works—addition, subtraction, multiplication, etc.
Now think of a human brain. When you make decisions, your mind processes thousands of experiences, emotions, and memories—but you can’t always explain exactly why you made a choice.
Modern AI models are more like the second case. Deep learning models, which power systems like ChatGPT, facial recognition, and medical diagnosis tools, learn from huge amounts of data and make complex decisions. But the exact way they reach those decisions? Hidden inside millions (or even billions) of parameters.
This lack of transparency creates major concerns in critical areas like:
Healthcare – If an AI predicts cancer in a patient, a doctor needs to understand why before starting treatment.
Hiring & Recruitment – If AI rejects a job applicant, was it due to skills or bias?
Finance – If AI denies a loan, should the bank trust the decision without an explanation?
Without clear explanations, AI decisions can be unfair, biased, or simply incorrect—without anyone noticing.
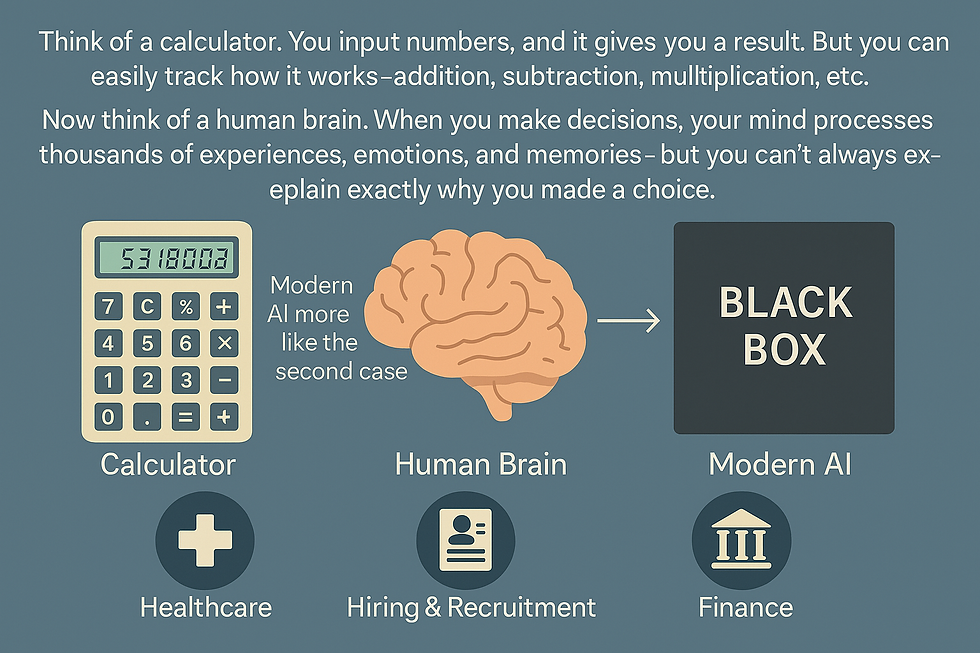
Why Should We Care?
Artificial Intelligence (AI) is making important decisions every day—who gets a loan, which candidate gets a job, or even how a self-driving car reacts on the road. But have you ever wondered, how does AI make these choices?
Right now, many AI systems work like a black box—they provide results, but we don’t always know why. And that’s a big problem because AI decisions impact real people in real ways.
If we don’t understand why AI makes certain choices, we can’t:
Fix errors when AI makes mistakes.
Ensure fairness so AI isn’t biased against anyone.
Trust AI in important fields like healthcare, finance, and hiring.
For example, would you trust a self-driving car if you didn’t know how it recognized pedestrians? Probably not! That’s why we need Explainable AI (XAI)—AI that shows its work instead of keeping it a secret.
What Is AI Interpretability & Why Does It Matter?
AI interpretability means understanding how AI makes decisions. It ensures that AI models are:
Fair – They don’t discriminate against certain groups.
Accountable – We can track mistakes and correct them.
Trustworthy – Users can rely on AI without blind faith.
For example:
Loan Approval – If AI decides who qualifies for a credit card, regulators must ensure the model isn’t biased against race, gender, or economic background.
Self-Driving Cars – If an AI misjudges an obstacle, engineers must understand why it made that mistake to prevent accidents.
Clearly, AI interpretability isn’t optional—it’s essential.
How Can We Make AI More Transparent?
Researchers are working on different methods to make AI more understandable:
Highlighting Important Factors
Just like a teacher underlines key points in a textbook, AI can highlight which data influenced its decision.
Example: If AI rejects a loan application, it should explain whether it was due to low income, poor credit score, or something else.
Using Explainable AI Tools (SHAP & LIME)
These tools break down AI decisions step by step, helping us see which inputs mattered the most.
Example: A medical AI that predicts heart disease can show whether age, weight, or cholesterol levels played a bigger role in the decision.
Building AI with Transparency from the Start
Instead of making AI that works like a mystery box, we should design it like a glass box—where every decision is visible and explainable.
This will help industries like healthcare, banking, and education make better and fairer AI models.
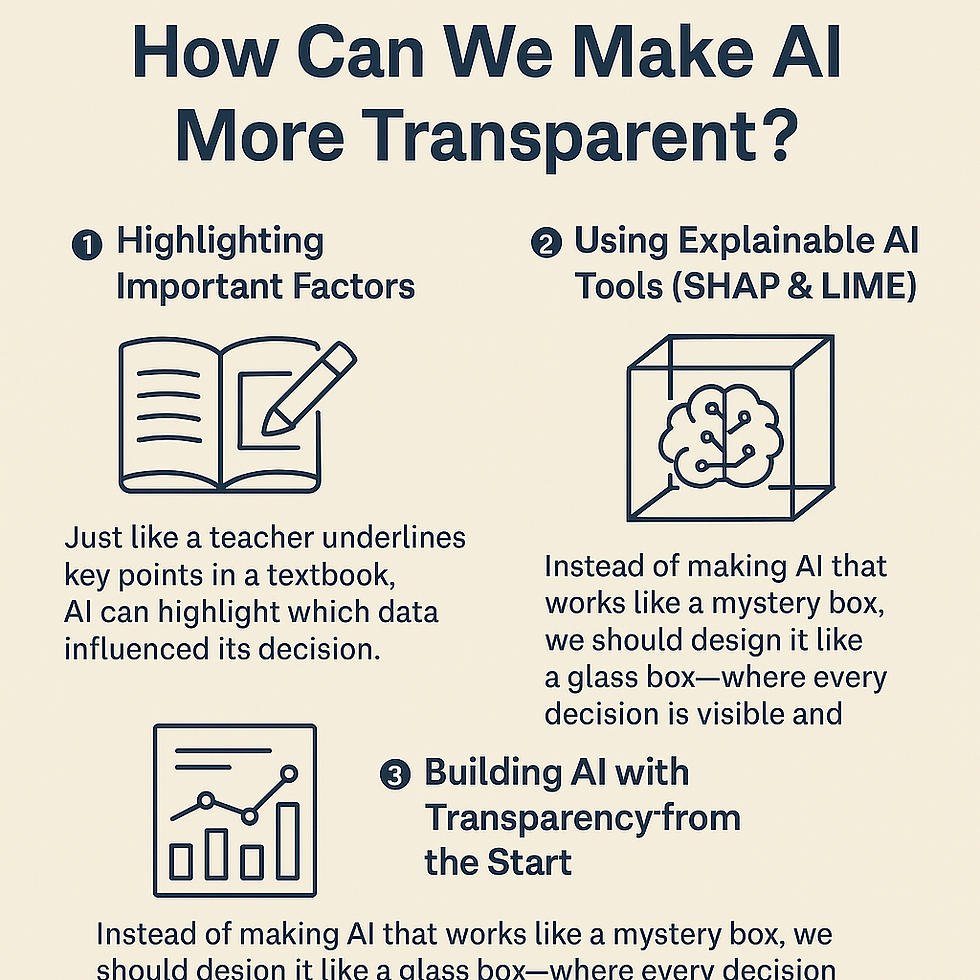
The Future of AI: More Transparency, More Trust
The world is moving towards more accountable AI. Governments are making rules to ensure AI is transparent, and researchers are finding ways to make AI easier to understand.
In the future, AI will not just be powerful but also explainable.
Instead of just getting answers, we’ll also get reasons behind those answers.
This will make AI more trustworthy and useful for everyone.
As learners and future AI users, we should:
Question AI decisions instead of blindly trusting them.
Learn about AI tools that explain decisions.
Support AI that is ethical and fair.
If AI is shaping our future, shouldn’t we understand how it works? What do you think? Should AI be forced to explain itself? Let’s discuss in the comments..!!
Commentaires